As was famously said by Richard Feynman in his last note: “What I cannot make, I cannot understand”. Over the last several decades, scanning transmission electron microscopy evolved to power atomic resolution imaging tools and also offer the advantage of an atomically confined interaction volume, allowing to induce atomic and molecular scale transformations in 2D materials, oxides, etc. However, these transformations are highly stochastic in nature, and their mechanisms are virtually unknown. We aim to harness the power of machine learning to understand the beam-induced transformations in solids and develop the pathways toward the experimental creation of materials with novel functionality. Fabrication of matter atom by atom on the atomic scale remains a long-held dream and one of the ultimate goals of nanotechnology. The need for such fabrication is by now well realized. In particular, quantum devices for large scale implementation of quantum computing, single spin magnetoelectronic devices, and scalable neuromorphic systems all need fabrication at the atomic level, including precise positioning of functional dopant atoms and avoiding even atomic-scale defects in the active region of the device and interconnects. Our vision for this field is summarized in several opinion pieces, including MRS Bulletin September 2017, Nature 539, 485 (2016), Nature 515, 487 (2014).
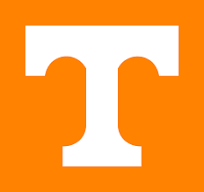